Professor Lee Jong-min from Hanyang University School of Electrical and Biomedical Engineering, and Professor Kim Bung-nyun from Seoul National University College of Medicine jointly announced the result of their joint research on January 17. They said they found out the way to accurately and rapidly diagnose autism spectrum disorder(ASD), which has difficulties in early diagnosis, using MRI neuroimaging-based objective¡¤biological indicators.
ASD is a neurodevelopmental disorder that occurs in 1~2% of children. The symptoms are having troubles in forming social relationships and emotional interaction, repetitive behaviors, and limited concentration. The cause of the disorder is known to be the result of interactions between genetic and environmental factors. Recently, more reports show that ASD is related to structure of the social brain and motor development disorders.
Until now, ASD were diagnosed through observing abnormal behaviors or expressions and symptom identification during developmental stages. Such methods have high conformity rates between experts, but there were limits in that the observer¡¯s subjectiveness might be involved and the cause of the disorder cannot be associated or identified. In this sense, it is emphasized to research on identifying the possibility of ADS diagnosis with objective and biological indicators.
Accordingly, the joint research team proceeded with research from May 2015 to September 2019 with 58 ASD patients and 48 control groups using MRI neuroimaging-based machine learning AI algorithm to evaluate diagnostic identification abilities. The age of research participants was 3~6 and only low-motor function patients(less than IQ 70) were included in the ASD group.
The machine learning algorithm was made into classifiers using machine learning such as the random forest. The classification three parameters ¡âT1 emphasis MRI imaging(quantitative measurement of the cerebral gray matter) ¡âdiffusion tensor imaging ¡âmultimodal MRI(T1 emphasis MRI and diffusion tensor imaging combined and measured) were used.
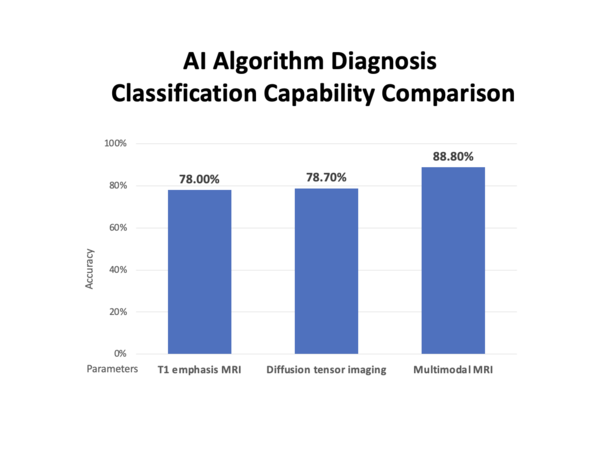
The research team separated the T1 emphasis MRI model, diffusion tensor imaging model, and multimodal MRI model according to parameters. Then, using a machine learning AI algorithm, they evaluated the diagnosis classification capabilities of separating into one of ASD or control groups. The multimodal MRI model showed 88.8% accuracy, 93.0% sensitivity, and 83.8% specification.
In particular, the accuracy of the multimodal MRI model was improved by 10%p compared to when the T1 emphasis MRI(78.0%) and the diffusion tensor imaging(78.7) were used independently. Furthermore, it was found that the most crucial imaging indicators of ASD diagnosis are the occipital lobe¡¯s cortical width, cerebellar peduncle¡¯s diffusion rate, and the back cingulate gyrus connection.
The joint research team emphasized that ¡°This research is meaningful in that the subjects were infant and child patients.¡± This is because there hasn¡¯t been lots of research in the neural imaging field as it was difficult to coordinate long MRI imaging hours for children of age 3~6, when most accurate and active treatments are needed.
Meanwhile, this research was published in the most recent edition of the international journal ¡®Journal of Autism and Developmental Disorders¡¯.